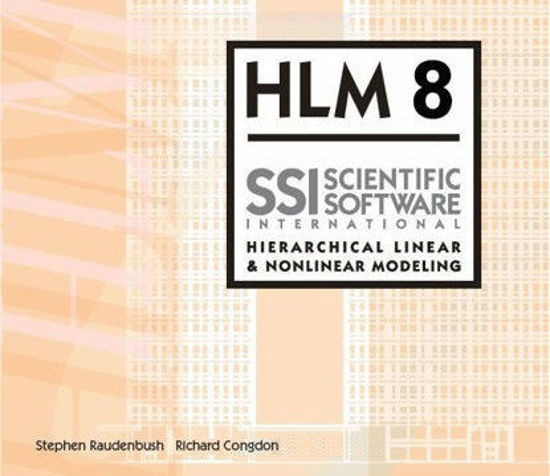
In social research and other fields, research data often have a hierarchical structure. That is, the individual subjects of study may be classified or arranged in groups which themselves have qualities that influence the study. It is clear that the analysis of such data requires specialized software. Hierarchical linear and nonlinear models (also called multilevel models) have been developed to allow for the study of relationships at any level in a single analysis, while not ignoring the variability associated with each level of the hierarchy.
HLM fits models to outcome variables that generate a linear model with explanatory variables that account for variations at each level, utilizing variables specified at each level. HLM not only estimates model coefficients at each level, but it also predicts the random effects associated with each sampling unit at every level.
HLM:
- can fit for two-, three- and four-level models with continuous, count, ordinal, and nominal outcome variables.
- is capable of fitting multivariate models where the variance at the lowest level of the hierarchy can assume a variety of forms/structures.
- offers a choice of three- and four-level nested models for cross-sectional and longitudinal models and four-way cross-classified and nested mixture models.
- allows the fitting of hierarchical models with dependent random effects (spatial design models).
- has the ability to estimate an HLM from incomplete data in the form of a completely automated approach that generates and analyses multiply imputed data sets from incomplete data. The model is fully multivariate and enables the analyst to strengthen imputation through auxiliary variables.
- includes the option to fit flexible combinations of Fixed Intercepts and Random Coefficients (FIRC) are now included in HLM2, HLM3, HLM4, HCM2, and HCM3.
- can analyze multiply-imputed/plausible value data.
- has an option to fit V-known models.